Random Forests®
Breiman 和 cutler 的 random forests
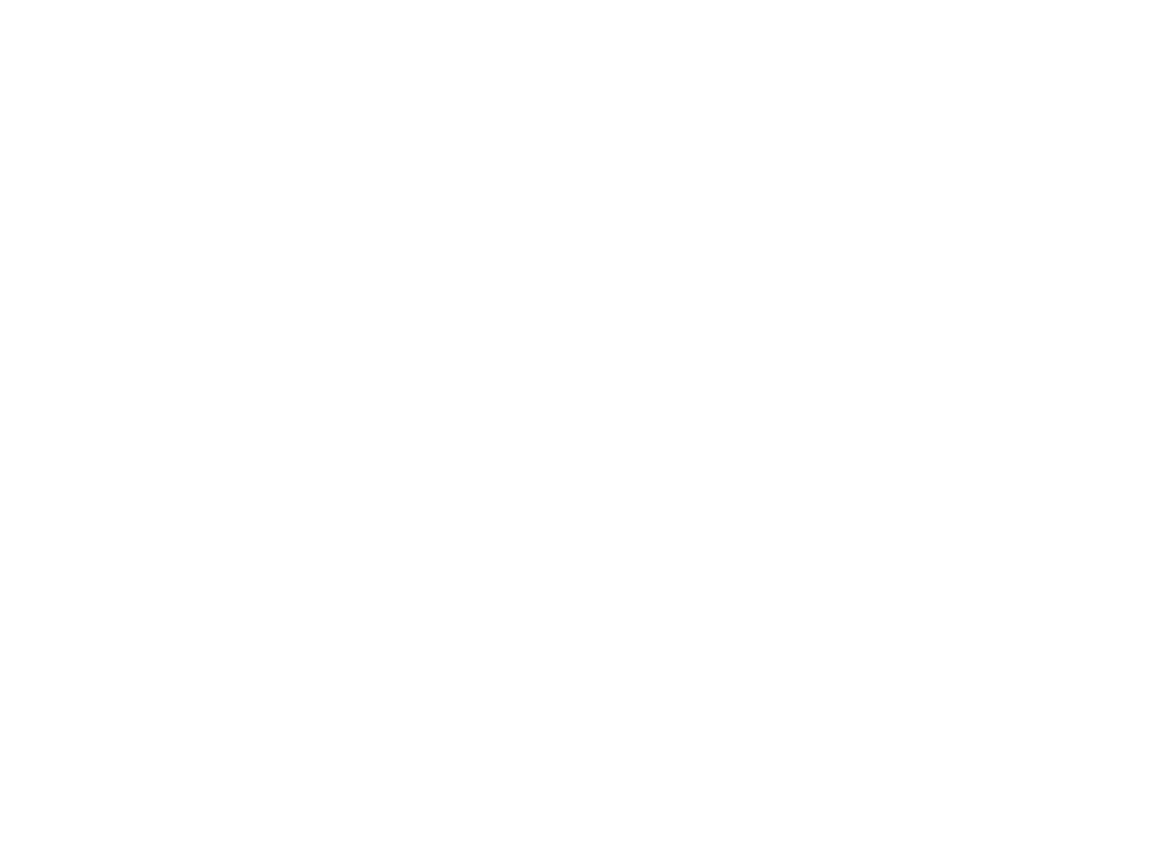
Random Forests® 建模引擎根据一系列分类和回归树 (CART®),对每个 CART 树所进行的预测值进行求和以确定森林的总体预测值,并同时确保决策树之间相互不受影响。
对于不熟悉 Random Forests 的用户来说,Random Forests 是由加州大学伯克利分校的 Leo Breiman 和 Adele Cutler 共同开发的强大集成技术,受到众多预测建模从业者的青睐。该算法看似简单,但可构建数百个独立的树,并利用来自观测值和变量的大量样本。
Random Forests 可以根据现成的数据评估无偏模型性能,有了这个独特功能,就无需单独的检验/验证样本。这使 Random Forests 立即成为广泛数据应用中的顶级预测建模工具,在这些应用中,变量的数量通常会超出可用观测值数量的好多倍。
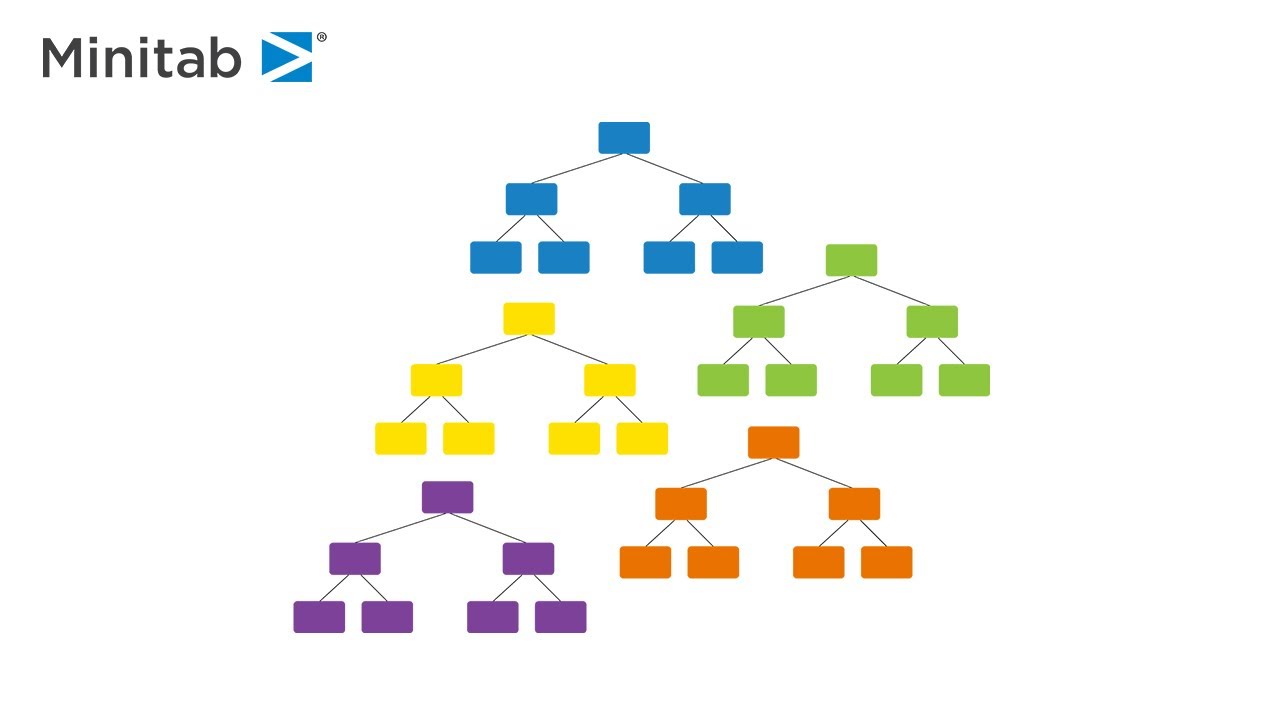
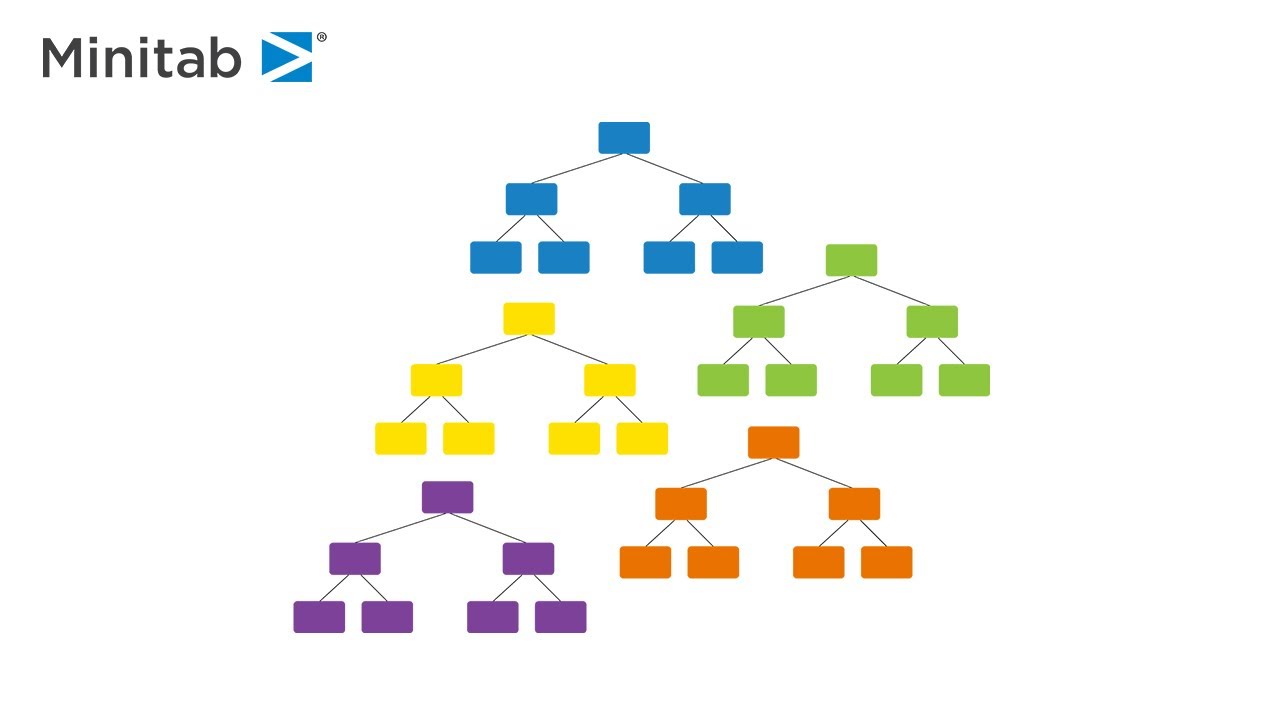
权责分明
Random Forests 具有一项独特功能,即利用数据集内的每条记录而不会陷入过度拟合危险。这对于小型(就观测值而言)数据集尤其重要,在此类数据集内,每条记录都可能会贡献有价值的内容。Random Forests 将确保模型中考虑了所有记录,并且不丢失任何见解。
强大的变量重要性
Random Forests 利用新颖的技术,根据预测变量的重要性对预测变量进行排名。当数据包含数千、数万甚至数十万变量或预测变量时,此功能非常方便,而这远远超出了传统的回归和分类工具的能力所及。Random Forests 可以处理这种极端情况,并报告要在后续研究中使用的变量。多轮抽样将提高这些见解的稳健性和质量。
是否有兴趣尝试使用最流行的建模引擎
Random Forests?
Minitab 的基于树的方法
无论您是刚刚起步还是希望将您的预测分析功能提升到新水平,Minitab 的基于树的建模引擎都将为您提供所需的功能。
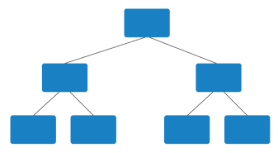
CART®
绝佳的分类树算法,为高级分析领域带来创新,开启了崭新的数据科学时代。
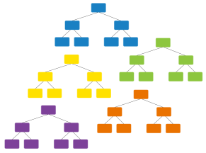
Random Forests®
能够方便地在一个位置利用多个备选分析、随机化策略和集成学习方法。
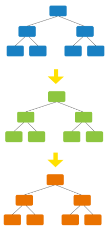
TreeNet®
最灵活、最强大的机器学习工具,能够按照统一的方式生成非常准确的模型。